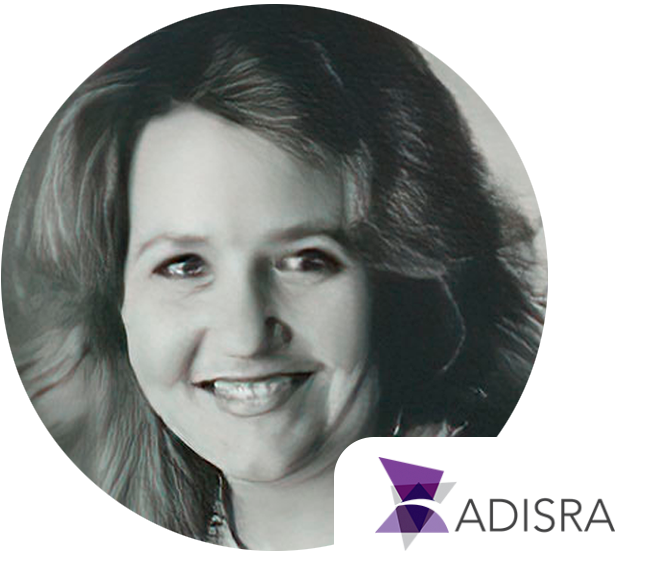
Bio
Marcia is an entrepreneur who has successfully grown a start-up from inception to a successful liquidity event. Prior to joining ADISRA, Marcia was the President of InduSoft, which was acquired by Invensys.
In the past, Marcia held a number of high tech senior management positions in the area of business development, strategy, competitive intelligence, marketing, and sales.
Marcia has more than 34 years’ experience in the software industry in diverse technology areas such as artificial intelligence, operating systems, rapid application development environment, output management, databases, directory services, data recovery, middleware, and industrial automation. Marcia is a contributing author in the book, “Client/Server Programming With RPC and DCE” as well as an author of many articles and whitepapers. Marcia holds a BS in Management Information System and Computer Science from Bowling Green State University and an Executive MBA from the University of New Hampshire.
Presentation
Evolution of Predictive Maintenance
Predictive maintenance has made enormous progress over the past several decades. General Electric (GE), in the 1980s, had a tool called GEN-X that was an expert system shell. It was used for troubleshooting and predictive maintenance for jet engines as well as GE consumer appliances. These expert systems incorporated domain-specific knowledge from existing troubleshooting manuals and enhanced by factory engineers with a background in engine performance analysis. Symbolic artificial intelligence, also known as expert systems, dominated diagnostic and predictive maintenance in that era. These expert systems were the precursor to condition-based maintenance that evolved to predictive maintenance. Predictive maintenance today uses machine learning to do a classification or regression approach to maintenance issues. This presentation will explore the evolution of predictive maintenance and how we can apply what we have learned to future applications in predictive maintenance.
Back
To the speakers page