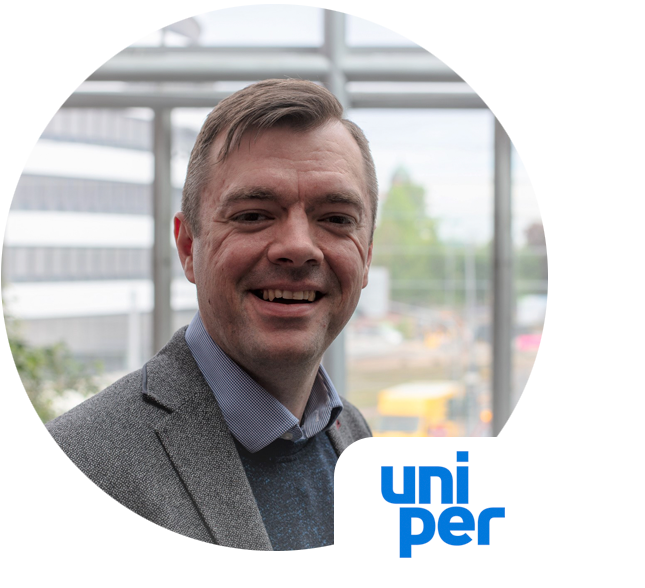
Pete Davies
Bio
Pete is a Dad, husband; proud dyslexic; and digital engineer. Working on tomorrows energy problems today, by combining engineering and digital technologies to create complete solutions to help the world in our energy transition to a lower carbon future. Pete has worked in the energy sector for 11+ years on the boundary between IT and engineering or trading delivering solutions for the opportunities and problems that arise in this space. Prior to the energy sector Pete worked in pharmaceuticals supporting the R&D and recipe aspects.
Pete is the founder of Enerlytics by Uniper, a digital platform which combines Uniper’s engineering expertise based on a pedigree of 100 years; owning and running 34GW of power plant with a drive for OEM independence.
Presentation
Artificial Intelligence and Machine Learning wizardry: how to overcome technical road blocks and make real for power generation
Artificial Intelligence (AI) and machine learning (ML) wizardry are often portrayed by the media, however there are some critical road blocks and a way to manage them in the power generation sector.
Road block 1: Low information learning, i.e. the ability to learn quickly from a small amount of information by calling on related information. Humans are amazing at this!
Road block 2: Your “CEO brain”. Humans have a filter system which does not let all thoughts reach your conscious (for good reason, some are pretty wild and dark!) and help keep your morality.
Road block 3: Power generation contains physical things which obey the laws of physics and these things are not your every day items, they reach extreme pressures and temperatures.
In an industry where health and safety rightfully leads the agenda we thankfully don’t have enough failures to utilise to use AI or ML appropriately.
Utilising 10+ years of advanced condition monitoring Uniper has recorded all faults detected and qualified that over time to create a database of “sudo failures” which is large enough to apply machine learning (ML) and artificial intelligence (AI) approaches with positive practical results.
Those 10+ years of sudo failures come from 50+ GW of assets, of all nearly all technology types, to create a unique knowledge base. This presentation and paper explains the process Uniper went through to deliver auto diagnosis (feature detection) for advanced condition monitoring and in some cases provide time to failure (prognosis).
Learnings:
Readers and attendees will learn:
- The limitation of Artificial Intelligence (AI) and machine learning (ML).
- How to manage and deliver with these limitations.
- What success looks like when you over come the limitations (case study).
Back
To the speakers page